How Can Statistics Transform Efficiency and Quality in a Manufacturing Plant?
In the fast-paced world of manufacturing, where precision and efficiency are paramount, the role of statistics cannot be overstated. As plants strive to optimize production processes, minimize waste, and enhance product quality, the power of statistical analysis emerges as a vital tool in their arsenal. By harnessing the insights derived from data, manufacturers can make informed decisions that not only streamline operations but also foster innovation and competitiveness in an ever-evolving market. This article delves into the multifaceted ways statistics can be applied within a manufacturing plant, illuminating the path to greater productivity and operational excellence.
Statistics serve as the backbone of decision-making in manufacturing environments, offering a systematic approach to understanding complex processes. From quality control to supply chain management, statistical methods empower teams to identify trends, monitor performance, and predict outcomes with remarkable accuracy. By analyzing data collected throughout the production cycle, manufacturers can pinpoint inefficiencies, reduce variability, and ensure that products meet stringent quality standards, ultimately leading to increased customer satisfaction and loyalty.
Moreover, the integration of statistical tools into manufacturing processes fosters a culture of continuous improvement. By employing techniques such as Six Sigma and Statistical Process Control (SPC), organizations can cultivate a proactive approach to problem-solving, enabling them to respond swiftly to challenges and capitalize on opportunities. As we
Quality Control and Improvement
Statistics play a critical role in quality control within manufacturing plants. By collecting and analyzing data from various stages of production, manufacturers can identify defects, monitor processes, and implement improvements. Techniques such as Statistical Process Control (SPC) utilize control charts to track variability and ensure processes remain within specified limits.
- Control charts help visualize data trends over time.
- Capability indices (Cp, Cpk) quantify how well a process meets specifications.
- Root cause analysis uses statistical methods to pinpoint sources of defects.
Implementing these techniques can lead to significant reductions in waste and rework, thereby enhancing overall product quality.
Inventory Management
Effective inventory management is crucial for maintaining production efficiency and minimizing costs. Statistical methods such as demand forecasting and inventory optimization can help manufacturers determine optimal stock levels, reorder points, and safety stock.
Statistical Method | Application |
---|---|
Time Series Analysis | Forecasting future demand based on historical data. |
ABC Analysis | Classifying inventory items based on their importance and value. |
Economic Order Quantity (EOQ) | Determining the optimal order quantity that minimizes total inventory costs. |
By applying these statistical methods, manufacturers can reduce holding costs and improve service levels.
Process Optimization
Statistical techniques are invaluable in optimizing manufacturing processes. Methods like Design of Experiments (DOE) allow engineers to assess how multiple factors impact product quality and production efficiency. By systematically varying inputs and measuring outputs, manufacturers can identify the most effective parameters.
- DOE can reduce trial-and-error testing time.
- Regression analysis helps in understanding relationships between variables.
- Simulation techniques provide insights into complex systems without physical trials.
These optimization strategies lead to enhanced productivity and reduced operational costs.
Predictive Maintenance
Predictive maintenance relies on statistical models to anticipate equipment failures before they occur. By analyzing historical maintenance data, manufacturers can predict when a machine is likely to fail and schedule maintenance accordingly. This proactive approach minimizes downtime and extends the lifespan of machinery.
- Reliability analysis assesses the likelihood of equipment failure.
- Time-to-failure distributions help in planning maintenance schedules.
- Statistical models can forecast maintenance needs based on usage patterns.
Integrating predictive maintenance strategies can lead to significant cost savings and improved operational efficiency.
Employee Performance Analysis
Statistics can also be applied to evaluate employee performance within a manufacturing plant. By analyzing productivity metrics, manufacturers can identify high performers, understand training needs, and improve workforce management.
- Performance metrics can include output per hour, error rates, and absenteeism.
- Statistical methods like regression analysis can uncover factors influencing performance.
- Surveys and feedback analysis can provide insights into employee satisfaction and engagement.
Utilizing these statistical insights enables manufacturers to foster a more productive and motivated workforce.
Quality Control and Improvement
Statistics play a critical role in maintaining and improving quality in manufacturing processes. By using statistical methods, manufacturers can detect variations in production quality, identify defects, and implement corrective actions.
- Statistical Process Control (SPC): This technique uses control charts to monitor manufacturing processes. Key benefits include:
- Early detection of process deviations
- Reduction of variability in production
- Improved product consistency
- Six Sigma: This data-driven approach aims to reduce defects and improve quality by identifying and removing causes of errors. It employs:
- DMAIC (Define, Measure, Analyze, Improve, Control) methodology
- Statistical tools such as hypothesis testing and regression analysis
Production Planning and Optimization
Statistical methods can significantly enhance production planning and scheduling. By analyzing historical data, manufacturers can forecast demand and optimize resource allocation.
- Demand Forecasting: Employing time series analysis and regression models helps predict future product demand based on historical sales data.
- Inventory Management: Statistics aid in determining optimal inventory levels through:
- Economic Order Quantity (EOQ) calculations
- Safety stock analysis based on variability in demand and lead times
Method | Purpose | Statistical Tools Used |
---|---|---|
Time Series Analysis | Demand Forecasting | ARIMA, Exponential Smoothing |
EOQ | Inventory Control | Basic EOQ Formula |
Safety Stock Analysis | Buffer Inventory | Variance and Standard Deviation |
Workforce Management
Effective workforce management is vital for maximizing productivity. Statistical analysis can help in determining optimal staffing levels and enhancing employee performance.
- Workload Analysis: By analyzing production data, managers can identify peak periods and adjust staffing accordingly.
- Performance Metrics: Key Performance Indicators (KPIs) like Overall Equipment Effectiveness (OEE) can be monitored statistically to evaluate employee productivity and machine efficiency.
Cost Analysis and Reduction
Manufacturers can utilize statistical methods to analyze costs and identify areas for potential savings.
- Cost Variance Analysis: By comparing actual costs to budgeted costs, manufacturers can determine variances and investigate their causes.
- Pareto Analysis: This technique helps identify the most significant factors contributing to costs, allowing for targeted interventions.
Supply Chain Management
Statistical methods are essential for optimizing supply chain operations, leading to increased efficiency and reduced costs.
- Vendor Performance Evaluation: Statistical techniques like control charts can monitor supplier performance over time, ensuring consistent quality.
- Lead Time Analysis: By employing regression analysis to study lead times, manufacturers can identify bottlenecks and optimize logistics processes.
Area of Focus | Statistical Method | Outcome |
---|---|---|
Supplier Evaluation | Control Charts | Quality Consistency |
Logistics Optimization | Regression Analysis | Reduced Lead Times |
Data-Driven Decision Making
The integration of statistical analysis into decision-making processes fosters a culture of data-driven decisions.
- Hypothesis Testing: Enables manufacturers to make informed decisions based on data analysis rather than intuition.
- Predictive Analytics: Utilizing machine learning algorithms and statistical models, manufacturers can anticipate future trends and make proactive adjustments in operations.
Leveraging Statistics for Enhanced Manufacturing Efficiency
Dr. Emily Chen (Industrial Statistician, Manufacturing Insights Journal). “Incorporating statistical process control (SPC) allows manufacturers to monitor production processes in real-time, enabling quick identification of deviations from quality standards. This proactive approach minimizes waste and enhances product consistency.”
James Patel (Operations Manager, Precision Parts Co.). “Utilizing statistical analysis for demand forecasting helps manufacturing plants optimize inventory levels. By accurately predicting customer demand, companies can reduce holding costs and improve service levels, ultimately leading to higher customer satisfaction.”
Linda Gomez (Quality Assurance Director, Global Manufacturing Solutions). “Statistical sampling techniques are essential in quality assurance processes. By applying these methods, manufacturers can efficiently assess product quality without the need for 100% inspection, thus saving time and resources while maintaining high standards.”
Frequently Asked Questions (FAQs)
How can statistics improve quality control in a manufacturing plant?
Statistics enable manufacturers to monitor production processes and identify variations that may affect product quality. By using statistical process control (SPC) techniques, plants can detect deviations from established standards, allowing for timely interventions to maintain quality.
What role do statistical analyses play in inventory management?
Statistical analyses help in forecasting demand, optimizing inventory levels, and reducing holding costs. Techniques such as regression analysis and time series analysis provide insights into inventory turnover rates and help in making informed decisions regarding stock replenishment.
How can statistical methods enhance production efficiency?
Statistical methods, such as lean manufacturing and Six Sigma, identify inefficiencies in production processes. By analyzing data on cycle times, throughput, and waste, manufacturers can implement process improvements that enhance overall efficiency and reduce costs.
What is the significance of statistical sampling in manufacturing?
Statistical sampling allows manufacturers to assess the quality of products without inspecting every item. By selecting a representative sample, manufacturers can make reliable inferences about the entire batch, saving time and resources while ensuring quality standards are met.
How can data visualization aid in the interpretation of manufacturing statistics?
Data visualization tools, such as charts and graphs, make complex statistical data more accessible and understandable. They help stakeholders quickly identify trends, patterns, and outliers, facilitating better decision-making based on statistical insights.
What are some common statistical tools used in manufacturing?
Common statistical tools include control charts, histograms, Pareto charts, and regression analysis. These tools assist in monitoring processes, analyzing data, and making informed decisions that drive continuous improvement in manufacturing operations.
In the context of a manufacturing plant, statistics play a crucial role in enhancing operational efficiency, quality control, and decision-making processes. By leveraging statistical methods, manufacturers can analyze production data, identify trends, and predict outcomes, which ultimately leads to improved productivity and reduced waste. The application of statistical tools such as control charts, process capability analysis, and regression analysis enables companies to monitor performance metrics and make informed adjustments to their processes.
Moreover, statistics facilitate the implementation of quality management systems, such as Six Sigma, by providing a framework for measuring and improving quality. Statistical sampling techniques allow manufacturers to conduct effective inspections without the need for exhaustive testing, thereby saving time and resources. Additionally, predictive analytics, grounded in statistical methodologies, empowers manufacturers to forecast demand and optimize inventory levels, ensuring that production aligns with market needs.
In summary, the integration of statistics into manufacturing operations not only enhances product quality but also drives continuous improvement initiatives. By embracing a data-driven approach, manufacturers can make strategic decisions that lead to operational excellence and competitive advantage in the marketplace. Ultimately, the effective use of statistics is a key component in achieving sustainable growth and profitability in the manufacturing sector.
Author Profile
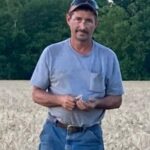
-
Hi, I’m Kendrik. This site is more than a blog to me. It’s a continuation of a promise.
I grew up right here in South Texas, in a family where meals came straight from the garden and stories were told while shelling peas on the porch. My earliest memories are of pulling weeds beside my grandfather, helping my mother jar pickles from cucumbers we grew ourselves, and learning, season by season, how to listen to the land.
Here at BrownsvilleFarmersMarket.com, I share what I’ve learned over the years not just how to grow crops, but how to nurture soil, nourish health, and rebuild food wisdom from the ground up. Whether you’re exploring composting, greenhouse farming, or hydroponic setups in your garage, I’m here to walk with you, row by row, one honest post at a time.
Latest entries
- April 26, 2025PlantsWhen Is the Best Time to Plant Cereal Rye for Deer?
- April 26, 2025PlantsDoes Wet And Forget Really Kill Plants? Unraveling the Truth Behind the Product!
- April 26, 2025HarvestingWhen Should You Harvest Your Yukon Gold Potatoes for Optimal Flavor?
- April 26, 2025Tree NurturingDo Palm Trees Have Big Roots? Exploring the Truth Behind Their Root Systems